Stochastic descent optimisation in Matlab
Using the Adam optimiser
21st February, 2017Seeking a minimum
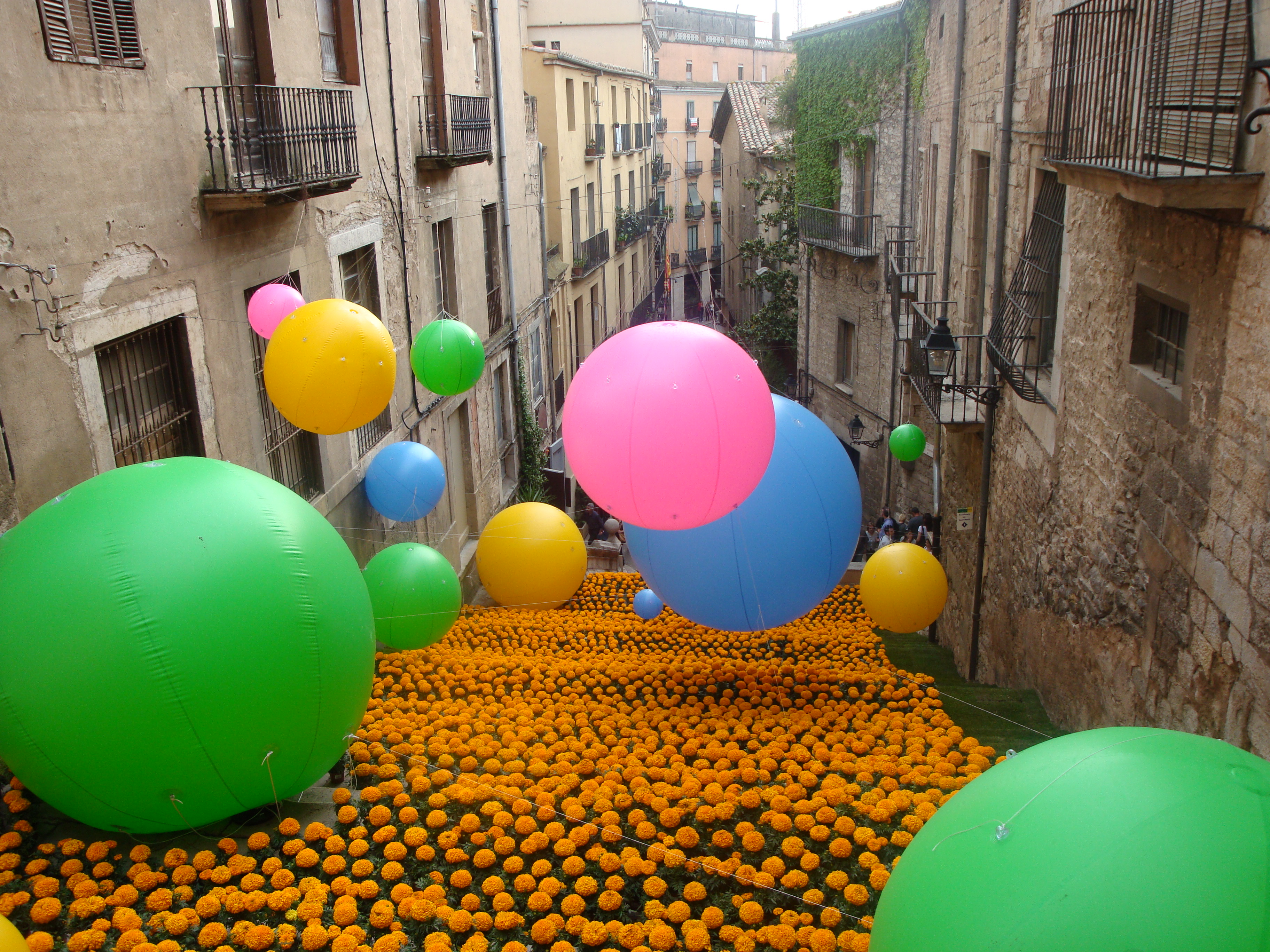
Seeking a minimum
Stochastic gradient descent is a powerful tool for optimisation, which relies on estimation of gradients over small, randomly-selected batches of data. This approach is efficient (since gradients only need to be evaluated over few data points at a time) and uses the noise inherent in the stochastic gradient estimates to help get around local minima. This is a Matlab
implementation of a recent powerful SGD algorithm.
The Adam optimiser from Kingma and Ba (2015) maintains estimates of the moments of the gradient independently for each parameter, with separate effective learning rates for each parameter. This gives the algorithm the benefits of accelerating over areas of the loss function with low-magnitude gradients; the ability to handle problems where individual parameters are poorly-scaled with respect to each other; and to push past small local minima in the loss function surface. Adam is designed to work on stochastic gradient descent problems; i.e. when only small batches of data are used to estimate the gradient on each iteration, or when stochastic dropout regularisation is used (Hinton et al. 2012).
Getting the code
Clone the git repository: github.
Usage
[x, fval, exitflag, output] = fmin_adam(fun, x0 <, stepSize, beta1, beta2, epsilon, nEpochSize, options>)
Examples
Simple regression problem with gradients
Set up a simple linear regression problem \( y = x\cdot\phi_1 + \phi_2 + \zeta \), where \(\zeta \sim N(0, 0.1) \). We'll take \( \phi = \left[3, 2\right] \) for this example. Let's draw some samples from this problem:
nDataSetSize = 1000; vfInput = rand(1, nDataSetSize); phiTrue = [3 2]; fhProblem = @(phi, vfInput) vfInput .* phi(1) + phi(2); vfResp = fhProblem(phiTrue, vfInput) + randn(1, nDataSetSize) * .1; plot(vfInput, vfResp, '.'); hold;

Now we define a cost function to minimise, which returns analytical gradients:
function [fMSE, vfGrad] = LinearRegressionMSEGradients(phi, vfInput, vfResp) % - Compute mean-squared error using the current parameter estimate vfRespHat = vfInput .* phi(1) + phi(2); vfDiff = vfRespHat - vfResp; fMSE = mean(vfDiff.^2) / 2; % - Compute the gradient of MSE for each parameter vfGrad(1) = mean(vfDiff .* vfInput); vfGrad(2) = mean(vfDiff); end
Initial parameters phi0
are Normally distributed. Call the fmin_adam
optimiser with a learning rate of 0.01
.
phi0 = randn(2, 1); phiHat = fmin_adam(@(phi)LinearRegressionMSEGradients(phi, vfInput, vfResp), phi0, 0.01) plot(vfInput, fhProblem(phiHat, vfInput), '.'); Output: Iteration Func-count f(x) Improvement Step-size ---------- ---------- ---------- ---------- ---------- 2130 4262 0.0051 5e-07 0.00013 ---------- ---------- ---------- ---------- ---------- Finished optimization. Reason: Function improvement [5e-07] less than TolFun [1e-06]. phiHat = 2.9498 2.0273

Linear regression with minibatches
Set up a simple linear regression problem, as above.
nDataSetSize = 1000; vfInput = rand(1, nDataSetSize); phiTrue = [3 2]; fhProblem = @(phi, vfInput) vfInput .* phi(1) + phi(2); vfResp = fhProblem(phiTrue, vfInput) + randn(1, nDataSetSize) * .1;
Configure minibatches. Minibatches contain random sets of indices into the data.
nBatchSize = 50; nNumBatches = 100; mnBatches = randi(nDataSetSize, nBatchSize, nNumBatches); cvnBatches = mat2cell(mnBatches, nBatchSize, ones(1, nNumBatches)); figure; hold; cellfun(@(b)plot(vfInput(b), vfResp(b), '.'), cvnBatches);

Define the function to minimise; in this case, the mean-square error over the regression problem. The iteration index nIter
defines which mini-batch to evaluate the problem over.
fhBatchInput = @(nIter) vfInput(cvnBatches{mod(nIter, nNumBatches-1)+1}); fhBatchResp = @(nIter) vfResp(cvnBatches{mod(nIter, nNumBatches-1)+1}); fhCost = @(phi, nIter) mean((fhProblem(phi, fhBatchInput(nIter)) - fhBatchResp(nIter)).^2);
Turn off analytical gradients for the adam
optimiser, and ensure that we permit sufficient function calls.
sOpt = optimset('fmin_adam'); sOpt.GradObj = 'off'; sOpt.MaxFunEvals = 1e4;
Call the fmin_adam
optimiser with a learning rate of 0.1
. Initial parameters are Normally distributed.
phi0 = randn(2, 1); phiHat = fmin_adam(fhCost, phi0, 0.1, [], [], [], [], sOpt)
The output of the optimisation process (which will differ over random data and random initialisations):
Iteration Func-count f(x) Improvement Step-size ---------- ---------- ---------- ---------- ---------- 711 2848 0.3 0.0027 3.8e-06 ---------- ---------- ---------- ---------- ---------- Finished optimization. Reason: Step size [3.8e-06] less than TolX [1e-05]. phiHat = 2.8949 1.9826
Detailed usage
Input arguments
fun
is a function handle [fCost <, vfCdX>] = @(x <, nIter>)
defining the function to minimise . It must return the cost at the parameter x
, optionally evaluated over a mini-batch of data. If analytical gradients are available (recommended), then fun
must return the gradients in vfCdX
, evaluated at x
(optionally over a mini-batch). If analytical gradients are not available, then complex-step finite difference estimates will be used.
To use analytical gradients (default), set options.GradObj = 'on'
. To force the use of finite difference gradient estimates, set options.GradObj = 'off'
.
fun
must be deterministic in its calculation of fCost
and vfCdX
, even if mini-batches are used. To this end, fun
can accept a parameter nIter
which specifies the current iteration of the optimisation algorithm. fun
must return estimates over identical problems for a given value of nIter
.
Steps that do not lead to a reduction in the function to be minimised are not taken.
Output arguments
x
will be a set of parameters estimated to minimise fCost
. fval
will be the value returned from fun
at x
.
exitflag
will be an integer value indicating why the algorithm terminated:
- 0: An output or plot function indicated that the algorithm should terminate.
- 1: The estimated reduction in 'fCost' was less than TolFun.
- 2: The norm of the current step was less than TolX.
- 3: The number of iterations exceeded MaxIter.
- 4: The number of function evaluations exceeded MaxFunEvals.
output
will be a structure containing information about the optimisation process:
.stepsize
— Norm of current parameter step.gradient
— Vector of current gradients evaluated atx
.funccount
— Number of calls tofun
made so far.iteration
— Current iteration of algorithm.fval
— Value returned byfun
atx
.exitflag
— Flag indicating reason that algorithm terminated.improvement
— Current estimated improvement infun
The optional parameters stepSize
, beta1
, beta2
and epsilon
are parameters of the Adam optimisation algorithm (see Kingma and Ba 2015). Default values of {1e-3, 0.9, 0.999, sqrt(eps)}
are reasonable for most problems.
The optional argument nEpochSize
specifies how many iterations comprise an epoch. This is used in the convergence detection code.
The optional argument options
is used to control the optimisation process (see optimset
). Relevant fields are:
.Display
.GradObj
.DerivativeCheck
.MaxFunEvals
.MaxIter
.TolFun
.TolX
.UseParallel
References
Diederik P. Kingma, Jimmy Ba. “Adam: A Method for Stochastic Optimization”, ICLR 2015. (arxiv).
Geoffrey E Hinton, Nitish Srivastava, Alex Krizhevsky, Ilya Sutskever, and Ruslan R. Salakhutdinov. 2012. “Improving neural networks by preventing co-adaptation of feature detectors.” arXiv preprint. (arxiv).